Unlocking Molecular Mysteries: Quantum Computing’s Role in Chemistry
The impact of quantum technology on chemistry is examined in this review, which also addresses existing issues, highlights developments in molecular modeling, and projects future paths for incorporating quantum computing into chemical research and industrial applications.
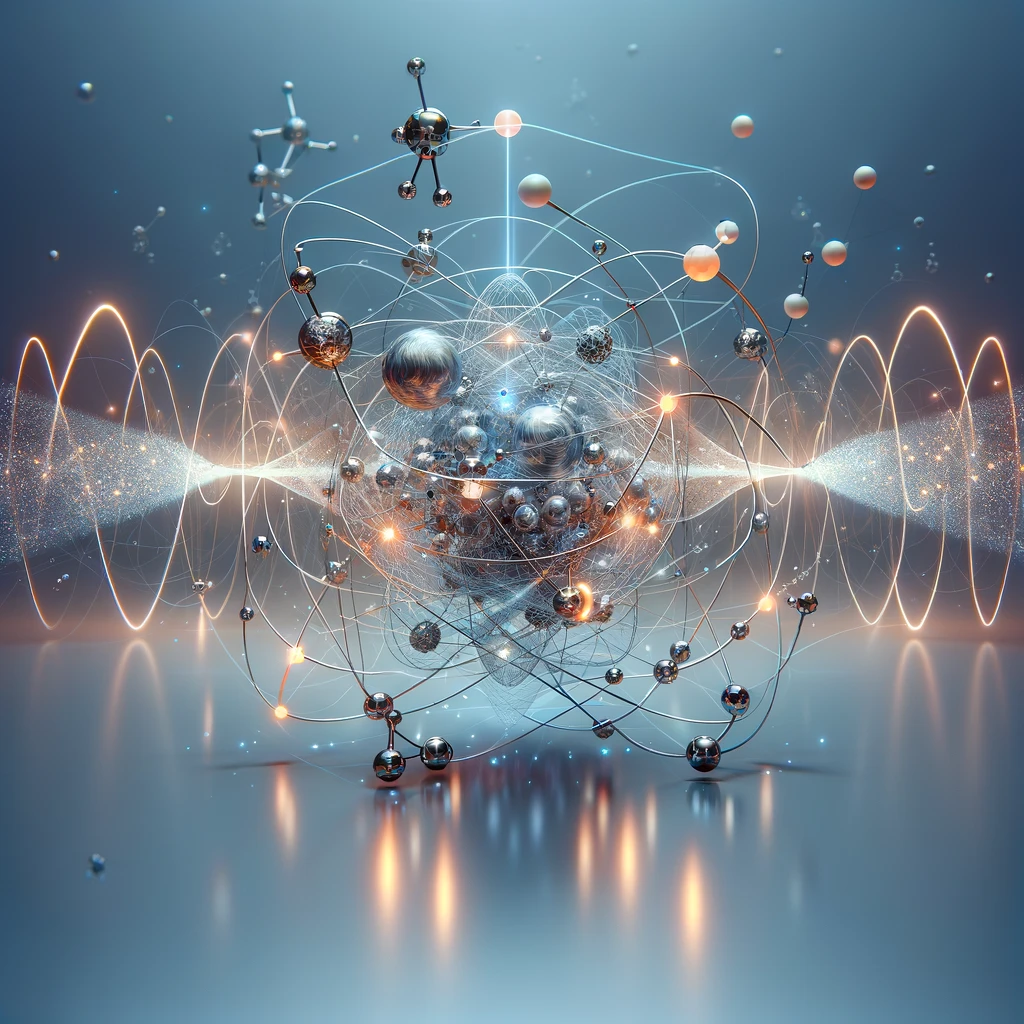
1. Introduction to Quantum Technology in Chemistry
The topic of quantum technology has gained great momentum and has the potential to transform other fields, including chemistry. The application of quantum concepts to industrial applications and chemical research has great potential for resolving hitherto unsolvable difficult issues. Numerous innovations, such as quantum computing and quantum simulations, are included in quantum technology, which opens up new ways to address chemistry-related problems (Gaita-Ariño et al., 2019).
Using molecule spins for quantum computation is a key component of quantum technology in chemistry. According to Gaita-Ariño et al. (2019), molecule nanomagnets with improved quantum coherence, like spin qubits, can be used to create molecular quantum units and construct quantum logic gates. This demonstrates how chemistry played a crucial part in creating and integrating molecular systems with distinct quantum features, which opened the door for quantum technology.
Furthermore, it has been suggested that utilizing the complementary strengths of quantum and classical resources can effectively reduce decoherence and identify excited states in quantum systems. Researchers hope to expand the capabilities of quantum-enabled computation by utilizing quantum devices backed by classical computer resources, opening up new avenues for solving challenging chemical issues (McClean et al., 2017).
Another promising avenue for the advancement of quantum chemistry is quantum plasmonics. Quantum plasmonics offers potential applications in single molecule sensing, quantum metrology, and quantum information processing through the manipulation of plasmonic gaps utilizing nanomaterials (Lee et al., 2021). This emphasizes how quantum technology is interdisciplinary, combining advances in quantum chemistry and nanophotonics to provide novel solutions.
Moreover, electronic structure Hamiltonian simulation has gained new insights from the development of quantum computing. Researchers can model intricate electronic structures with previously unheard-of efficiency by utilizing quantum computers, which presents a way to advance quantum chemistry (Whitfield et al., 2011). This is an example of how understanding and forecasting chemical events might significantly advance as a result of the convergence of quantum technology and chemistry.
There is a great deal of potential for revolutionizing both research and industrial applications when quantum technology is included into chemistry. Through the utilization of quantum plasmonics, hybrid quantum-classical methods, molecular spins, and quantum simulations, scientists are leading the way in a new era of quantum principles driving innovation in chemistry. Quantum technologies have the potential to completely transform the chemical field as they develop further, providing hitherto unheard-of possibilities for resolving intricate chemical issues and expanding scientific understanding.
2. Applications of Quantum Computing in Molecular Modeling
In molecular modeling, quantum computing has emerged as a potent tool that offers remarkable accuracy in simulating molecular structures, reaction kinetics, and energy landscapes. Materials science and drug development have advanced as a result of researchers’ increased accuracy in examining the complex features of molecular systems thanks to quantum computing, which makes use of the ideas of quantum mechanics (Li et al., 2022).
Advances in quantum computing have demonstrated great potential in chemical property prediction, transforming drug development, material design, and catalyst optimization applications (Li et al., 2022). Scientists can explore new chemical regions and assess possible treatment options with unprecedented efficiency by using quantum algorithms and simulations (Sullivan et al., 2015).
The application of quantum machine learning algorithms to drug development expands the potential applications of quantum computing. These cutting-edge methods open up new possibilities for investigating intricate chemical regions and improving medication prospects in addition to increasing the precision of molecular modeling (Batra et al., 2021).
Furthermore, the predictive power of quantum chemistry in the development of pharmaceutical processes has been highlighted, illustrating the influence of quantum computations on catalyst design and reaction optimization studies (Lam et al., 2020). In the pharmaceutical industry, quantum chemistry is essential for providing understanding of molecular interactions and directing experimental efforts.
Moreover, quantum generative adversarial networks’ application in generative chemistry demonstrates the variety of ways that quantum computing may be used to explore chemical regions and create new kinds of molecules (Kao et al., 2023). Researchers can broaden the scope of computational chemistry by using quantum algorithms to open up new avenues for combinatorial optimization and drug development.
The application of quantum computing to molecular modeling is a noteworthy development in chemistry. Quantum computing offers unmatched accuracy and efficiency in drug development and materials science, from accurate simulations of molecular structures to the investigation of unexplored chemical regions. As quantum technologies develop, they present enormous promise for revolutionizing the field of molecular modeling and accelerating chemical research.
3. Challenges and Future Perspectives
While there are many benefits to quantum technology in chemistry, there are also obstacles that need to be overcome before it can be fully included into conventional chemical research. One major drawback is the hardware limitations; many quantum algorithms are too complex for many quantum technologies, both near-term and current, to operate efficiently (McClean et al., 2017). This hardware constraint prevents quantum computing from reaching its full potential in chemistry, and further developments in quantum hardware are required to make complex algorithms for molecular modeling run more smoothly.
Moreover, algorithmic developments are critical to overcome the obstacles that quantum chemistry faces. Current computational approaches are severely limited by the lack of computationally efficient ways for simulating quantum systems on classical computers (Whitfield et al., 2011). Improving the capabilities of quantum chemistry simulations and enabling the precise modeling of molecule structures and reaction dynamics depend on the development of algorithms that can accurately simulate electronic structure Hamiltonians on quantum computers.
In order to effectively address these issues, future paths for research in quantum technology for chemistry should prioritize interdisciplinary collaboration. Through bringing together specialists from many disciplines, including computer science, chemistry, and quantum physics, scientists can work toward creating hybrid quantum-classical hierarchies that reduce decoherence and improve the identification of excited states in quantum systems (McClean et al., 2017). The advancement of quantum computing in chemistry and the realization of its revolutionary promise in the resolution of challenging chemical issues depend heavily on this cooperative approach.
In summary, although quantum technology has enormous potential to transform chemistry, its successful integration into traditional chemical research will depend on how well existing issues like hardware restrictions and algorithmic difficulties are resolved. The widespread use of quantum computing in chemistry can be facilitated by researchers by encouraging interdisciplinary collaboration and focusing on developments in quantum hardware and algorithms. This will lead to breakthroughs in molecular modeling, materials science, and drug discovery.
References:
Batra, K., Zorn, K., Foil, D., Minerali, E., Gawriljuk, V., Lane, T., … & Ekins, S. (2021). Quantum machine learning algorithms for drug discovery applications. Journal of Chemical Information and Modeling, 61(6), 2641-2647. https://doi.org/10.1021/acs.jcim.1c00166
Gaita‐Ariño, A., Luìs, F., Hill, S., & Coronado, E. (2019). Molecular spins for quantum computation. Nature Chemistry, 11(4), 301-309. https://doi.org/10.1038/s41557-019-0232-y
Kao, P., Yang, Y., Chiang, W., Hsiao, J., Cao, Y., Aliper, A., … & Lin, Y. (2023). Exploring the advantages of quantum generative adversarial networks in generative chemistry. Journal of Chemical Information and Modeling, 63(11), 3307-3318. https://doi.org/10.1021/acs.jcim.3c00562
Lam, Y., Abramov, Y., Ananthula, R., Elward, J., Hilden, L., Lill, S., … & Tanoury, G. (2020). Applications of quantum chemistry in pharmaceutical process development: current state and opportunities. Organic Process Research & Development, 24(8), 1496-1507. https://doi.org/10.1021/acs.oprd.0c00222
Lee, J., Jeon, D., & Yeo, J. (2021). Quantum plasmonics: energy transport through plasmonic gap. Advanced Materials, 33(47). https://doi.org/10.1002/adma.202006606
Li, W., Huang, Z., Cao, C., Huang, Y., Shuai, Z., Sun, X., … & Lv, D. (2022). Toward practical quantum embedding simulation of realistic chemical systems on near-term quantum computers. Chemical Science, 13(31), 8953-8962. https://doi.org/10.1039/d2sc01492k
McClean, J., Schwartz, M., Carter, J., & Jong, W. (2017). Hybrid quantum-classical hierarchy for mitigation of decoherence and determination of excited states. Physical Review A, 95(4). https://doi.org/10.1103/physreva.95.042308
McClean, J., Schwartz, M., Carter, J., & Jong, W. (2017). Hybrid quantum-classical hierarchy for mitigation of decoherence and determination of excited states. Physical Review A, 95(4). https://doi.org/10.1103/physreva.95.042308
Sullivan, D., Liu, Y., Mott, B., Kaludov, N., & Martinov, M. (2015). Discovery of novel liver-stage antimalarials through quantum similarity. Plos One, 10(5), e0125593. https://doi.org/10.1371/journal.pone.0125593
Whitfield, J., Biamonte, J., & Aspuru‐Guzik, A. (2011). Simulation of electronic structure hamiltonians using quantum computers. Molecular Physics, 109(5), 735-750. https://doi.org/10.1080/00268976.2011.552441
Whitfield, J., Biamonte, J., & Aspuru‐Guzik, A. (2011). Simulation of electronic structure hamiltonians using quantum computers. Molecular Physics, 109(5), 735-750. https://doi.org/10.1080/00268976.2011.552441