Innovations in Automated Synthesis Technologies
This paper examines how automated synthesis has transformed the field of chemistry, stressing recent developments, increased productivity, and upcoming difficulties. It highlights the room for creativity in reaction optimization and research.
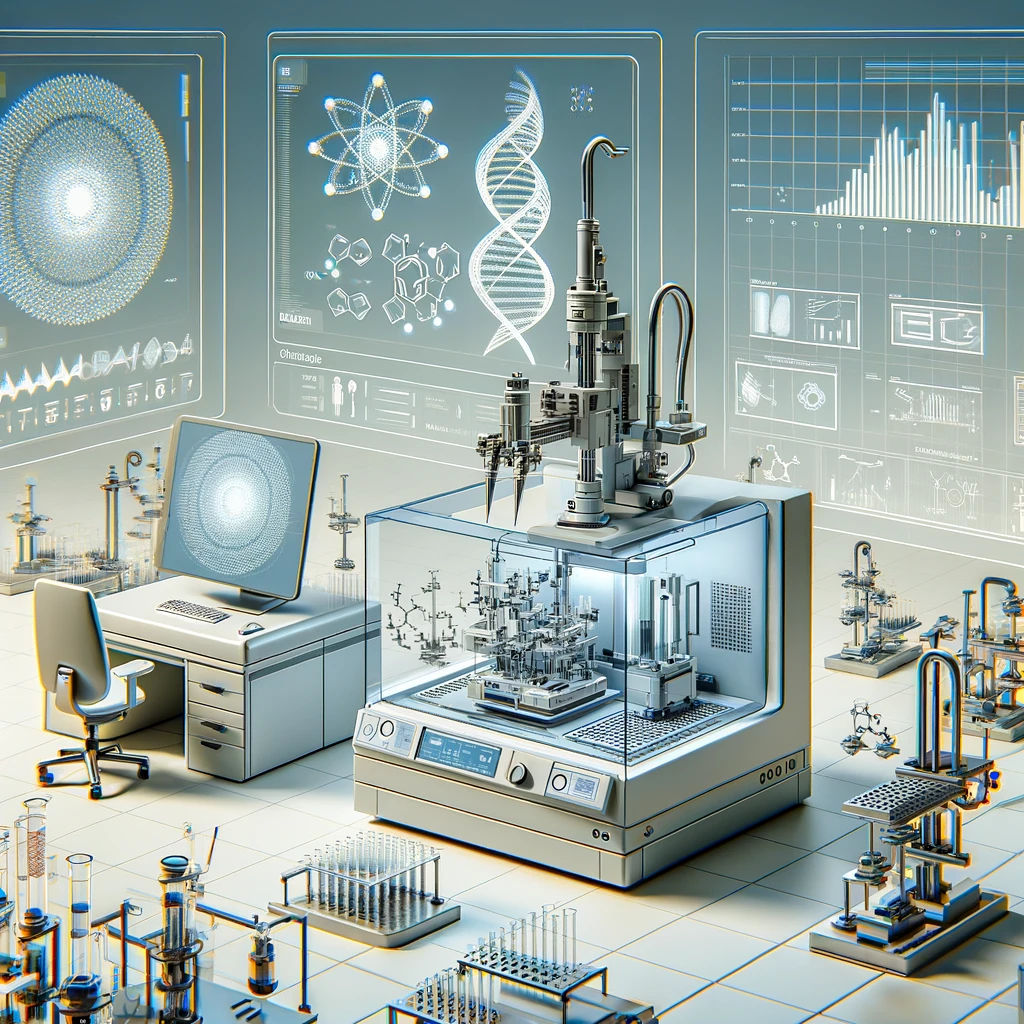
1. Introduction to Automated Synthesis
Chemical automated synthesis refers to the process of proposing and carrying out synthetic routes through the use of robotic platforms and artificial intelligence algorithms (Empel & Koenigs, 2019). This development offers advantages including improved efficiency, accuracy, and repeatability in chemical synthesis by marking a substantial transition from manual synthesis methods to automated processes (Panza et al., 2018). Nanotechnology, miniaturization, and the creation of self-driving labs have all contributed to the shift towards automation (Mattes et al., 2019; Soldatov et al., 2021). The synthesis of oligosaccharides, biomolecules, and functional materials are only a few of the applications for which these technologies have improved the speed and accuracy of synthesis (Mattes et al., 2019; Soldatov et al., 2021).
Chemical synthesis has undergone a revolution thanks to automation, which makes it possible to synthesize a huge number of chemicals that were previously impractical to do by hand (Ghose et al., 1998). According to Haji (2016), multicomponent reactions (MCRs) have become an essential procedure for effectively producing complex chemical molecules. Furthermore, the standardization of chemical informatics brought about by synthesis automation has improved access to complicated compounds and repeatability by facilitating the smooth transfer of digital synthesis codes between platforms (Steiner et al., 2019; Hammer et al., 2021).
Furthermore, the automation of peptide synthesis has greatly decreased the requirement for human intervention, increasing the accuracy and efficiency of the synthesis of lengthy peptides (Tian et al., 2022). Additionally, automation has been crucial in the creation of new techniques for bond formation and reaction optimization, which has sped up the search for new compounds (Pijper et al., 2023; Chen, 2023). Chemical synthesis has been made more efficient by the incorporation of automation, which has also made it possible to find functional materials and possible medication candidates more quickly (Pennington, 2024; Baranczak et al., 2017).
Chemical synthesis is now more efficient, precise, and reproducible thanks to the shift from human to automated procedures. This has completely changed modern chemistry. In addition to speeding up the synthesis of complicated molecules, the combination of robots, digital labware, and artificial intelligence has broadened the range of applications in a number of disciplines, including drug discovery, material research, and nanotechnology. Chemical synthesis automation signifies a paradigm change toward a more creative and effective approach to molecule design and discovery.
2. Technologies in Automated Synthesis
Key technologies like robotic systems, flow chemistry, and machine learning algorithms have greatly increased automated synthesis in chemistry (Gromski et al., 2020; Wilbraham et al., 2020; Yu & Huang, 2021). Robotic systems have made it possible to automate a number of synthesis procedures, such as reaction monitoring and reagent distribution, which has streamlined the process and reduced the need for human intervention (Gromski et al., 2020; Wilbraham et al., 2020). By enabling continuous reactions, flow chemistry has transformed synthesis by improving scalability and controlling reaction parameters (Gromski et al., 2020; Wilbraham et al., 2020). Chemical reaction discovery and optimization have been sped up thanks in large part to machine learning algorithms’ ability to anticipate reaction outcomes, optimize reaction conditions, and even design new compounds (Yu & Huang, 2021).
Chemical reaction discovery, optimization, and scalability have been made easier by the incorporation of these technologies into synthesis processes. High precision and repeatability reactions can be carried out by robotic systems with sensors for real-time monitoring, which reduces experimental variability and produces consistent results (Gromski et al., 2020; Wilbraham et al., 2020). Rapid reaction optimization is made possible by flow chemistry systems, which permit the systematic and continuous adjustment of reaction parameters. This process identifies the ideal circumstances while consuming the least amount of time and resources (Gromski et al., 2020; Wilbraham et al., 2020). Chemical reaction discovery and optimization can be accelerated by the use of machine learning algorithms that analyze large datasets of chemical reactions to predict reaction outcomes, suggest new paths for reactions, and improve reaction settings (Yu & Huang, 2021).
Moreover, the integration of these technologies has made it possible to create autonomous synthesis platforms that require little assistance from humans to complete complicated synthesis tasks. According to studies by Gromski et al. (2020), Wilbraham et al. (2020), Yu & Huang (2021), and others, these platforms have the ability to autonomously plan and carry out synthetic routes, analyze reaction outcomes in real-time, and iteratively optimize reaction conditions based on data from sensors and machine learning models. In addition to improving chemical synthesis’s efficiency and reproducibility, this integration of robotic systems, flow chemistry, and machine learning algorithms has sped up the search for novel compounds and materials with a variety of uses in material science, drug discovery, and other fields.
The integration of robotic systems, flow chemistry, and machine learning algorithms has transformed automated synthesis in the field of chemistry, facilitating the swift identification, enhancement, and expansion of chemical reactions. Chemical synthesis has seen innovation as a result of these technologies, which have opened the door for the creation of autonomous synthesis platforms capable of carrying out challenging synthesis operations with extreme precision and efficiency.
3. Challenges and Future Directions
Many obstacles prevent automated synthesis in chemistry from being widely used and from being as effective as it may be. These difficulties include the complexity of fully integrated platforms, the constraints of generic synthetic methods for routine synthesis, and the adaptability to various chemical reactions (Wen et al., 2018; Gioiello et al., 2020; Chapman et al., 2016). One major obstacle to automated synthesis is the lack of generic synthetic methods for routine synthesis, especially in complex compounds like glycans (Wen et al., 2018). Additionally, only top academic research groups and pharmaceutical companies are currently able to practically realize fully integrated platforms, highlighting the difficulty and expense of automating synthesis processes (Gioiello et al., 2020). Furthermore, the oxygen sensitivity and sensitivity of some processes (controlled radical polymerizations, for example) limit the exploration of a broad range of structures for different applications and complicate combinatorial synthesis (Chapman et al., 2016).
Despite these difficulties, new developments in science and technology provide encouraging ways to go above the obstacles that automated synthesis faces. Push-button automated multistep syntheses of active pharmaceutical components are now possible thanks to the integration of solid-phase synthesis with continuous-flow operation, which addresses shortcomings in conventional synthesis methods (Liu et al., 2021). Additionally, improvements in artificial intelligence and machine learning algorithms are improving the ability to forecast reaction results and optimize reaction circumstances, which increases the effectiveness and adaptability of automated synthesis processes (Schrier, 2023). One potential solution to the complexity and financial constraints of automated synthesis is the creation of autonomous synthesis platforms, which are able to plan, carry out, and optimize synthetic routes with little to no human interaction (Seifrid et al., 2022).
Prospects for the future of automated synthesis also include the creation of more automated and generalized methods for producing small molecules. This could change the emphasis from synthesis to function, allowing for the investigation of the characteristics of pure and well-defined compounds on a chemical level (Li et al., 2015). Furthermore, more effective and scalable synthesis procedures are being made possible by developments in high-throughput synthesis techniques, such as the automation of glycan assembly and the synthesis of protein microarrays (Kumar et al., 2017; Li et al., 2020). Automated synthesis is set to become more widely used and stimulate innovation in chemical synthesis by overcoming constraints and obstacles using creative technology and approaches.
References:
Baranczak, A., Tu, N., Marjanovic, J., Searle, P., Vasudevan, A., & Djurić, S. (2017). Integrated platform for expedited synthesis–purification–testing of small molecule libraries. Acs Medicinal Chemistry Letters, 8(4), 461-465. https://doi.org/10.1021/acsmedchemlett.7b00054
Chapman, R., Gormley, A., Stenzel, M., & Stevens, M. (2016). Combinatorial low‐volume synthesis of well‐defined polymers by enzyme degassing. Angewandte Chemie, 128(14), 4576-4579. https://doi.org/10.1002/ange.201600112
Chen, J. (2023). Wireless electrochemical reactor for accelerated exploratory study of electroorganic synthesis. Acs Central Science, 9(9), 1820-1826. https://doi.org/10.1021/acscentsci.3c00856
Empel, C. and Koenigs, R. (2019). Artificial‐intelligence‐driven organic synthesis—en route towards autonomous synthesis?. Angewandte Chemie, 58(48), 17114-17116. https://doi.org/10.1002/anie.201911062
Ghose, A., Viswanadhan, a., & Wendoloski, J. (1998). A knowledge-based approach in designing combinatorial or medicinal chemistry libraries for drug discovery. 1. a qualitative and quantitative characterization of known drug databases. Journal of Combinatorial Chemistry, 1(1), 55-68. https://doi.org/10.1021/cc9800071
Gioiello, A., Piccinno, A., Lozza, A., & Cerra, B. (2020). The medicinal chemistry in the era of machines and automation: recent advances in continuous flow technology. Journal of Medicinal Chemistry, 63(13), 6624-6647. https://doi.org/10.1021/acs.jmedchem.9b01956
Gromski, P., Granda, J., & Cronin, L. (2020). Universal chemical synthesis and discovery with ‘the chemputer’. Trends in Chemistry, 2(1), 4-12. https://doi.org/10.1016/j.trechm.2019.07.004
Haji, M. (2016). Multicomponent reactions: a simple and efficient route to heterocyclic phosphonates. Beilstein Journal of Organic Chemistry, 12, 1269-1301. https://doi.org/10.3762/bjoc.12.121
Hammer, A., Leonov, A., Bell, N., & Cronin, L. (2021). Chemputation and the standardization of chemical informatics. Jacs Au, 1(10), 1572-1587. https://doi.org/10.1021/jacsau.1c00303
Kumar, N., Schumacher, F., Hahm, H., & Seeberger, P. (2017). Pushing the limits of automated glycan assembly: synthesis of a 50mer polymannoside. Chemical Communications, 53(65), 9085-9088. https://doi.org/10.1039/c7cc04380e
Li, B., Zhang, Y., Wang, Y., Yang, X., & Nie, X. (2020). Rapid fabrication of protein microarrays via autogeneration and on-chip purification of biotinylated probes. Acs Synthetic Biology, 9(9), 2267-2273. https://doi.org/10.1021/acssynbio.0c00343
Li, J., Grillo, A., & Burke, M. (2015). From synthesis to function via iterative assembly of n-methyliminodiacetic acid boronate building blocks. Accounts of Chemical Research, 48(8), 2297-2307. https://doi.org/10.1021/acs.accounts.5b00128
Liu, C., Xie, J., Wu, W., Wang, M., Chen, W., Idres, S., … & Wu, J. (2021). Automated synthesis of prexasertib and derivatives enabled by continuous-flow solid-phase synthesis. Nature Chemistry, 13(5), 451-457. https://doi.org/10.1038/s41557-021-00662-w
Mattes, D., Jung, N., Weber, L., Bräse, S., & Breitling, F. (2019). Miniaturized and automated synthesis of biomolecules—overview and perspectives. Advanced Materials, 31(26). https://doi.org/10.1002/adma.201806656
Panza, M., Pistorio, S., Stine, K., & Demchenko, A. (2018). Automated chemical oligosaccharide synthesis: novel approach to traditional challenges. Chemical Reviews, 118(17), 8105-8150. https://doi.org/10.1021/acs.chemrev.8b00051
Pennington, L. (2024). Total synthesis as training for medicinal chemistry. Acs Medicinal Chemistry Letters, 15(2), 156-158. https://doi.org/10.1021/acsmedchemlett.3c00556
Pijper, B., Abdiaj, I., Leonori, D., & Alcázar, J. (2023). Development of an automated platform for c(sp3)−c(sp3) bond formation via xat chemistry. Chemcatchem, 15(4). https://doi.org/10.1002/cctc.202201289
Schrier, J. (2023). In pursuit of the exceptional: research directions for machine learning in chemical and materials science. Journal of the American Chemical Society, 145(40), 21699-21716. https://doi.org/10.1021/jacs.3c04783
Seifrid, M., Pollice, R., Aguilar‐Granda, A., Chan, Z., Hotta, K., Ser, C., … & Aspuru‐Guzik, A. (2022). Autonomous chemical experiments: challenges and perspectives on establishing a self-driving lab. Accounts of Chemical Research, 55(17), 2454-2466. https://doi.org/10.1021/acs.accounts.2c00220
Soldatov, M., Butova, V., Pashkov, D., Butakova, M., Medvedev, P., Чернов, А., … & Солдатов, А. (2021). Self-driving laboratories for development of new functional materials and optimizing known reactions. Nanomaterials, 11(3), 619. https://doi.org/10.3390/nano11030619
Steiner, S., Wolf, J., Glatzel, S., Andreou, A., Granda, J., Keenan, G., … & Cronin, L. (2019). Organic synthesis in a modular robotic system driven by a chemical programming language. Science, 363(6423). https://doi.org/10.1126/science.aav2211
Tian, J., Li, Y., Ma, B., & Shang, S. (2022). Automated peptide synthesizers and glycoprotein synthesis. Frontiers in Chemistry, 10. https://doi.org/10.3389/fchem.2022.896098
Wen, L., Edmunds, G., Gibbons, C., Zhang, J., Gadi, M., Zhu, H., … & Wang, P. (2018). Toward automated enzymatic synthesis of oligosaccharides. Chemical Reviews, 118(17), 8151-8187. https://doi.org/10.1021/acs.chemrev.8b00066
Wilbraham, L., Mehr, S., & Cronin, L. (2020). Digitizing chemistry using the chemical processing unit: from synthesis to discovery. Accounts of Chemical Research, 54(2), 253-262. https://doi.org/10.1021/acs.accounts.0c00674
Yu, Z. and Huang, W. (2021). Accelerating optimizing the design of carbon‐based electrocatalyst via machine learning. Electroanalysis, 34(4), 599-607. https://doi.org/10.1002/elan.202100224