Frontier of Computational and Theoretical Chemistry
This study examines the evolution, current methodology, and future directions of theoretical and computational chemistry, focusing on how these approaches transform our understanding and prediction of chemical behaviors and characteristics.
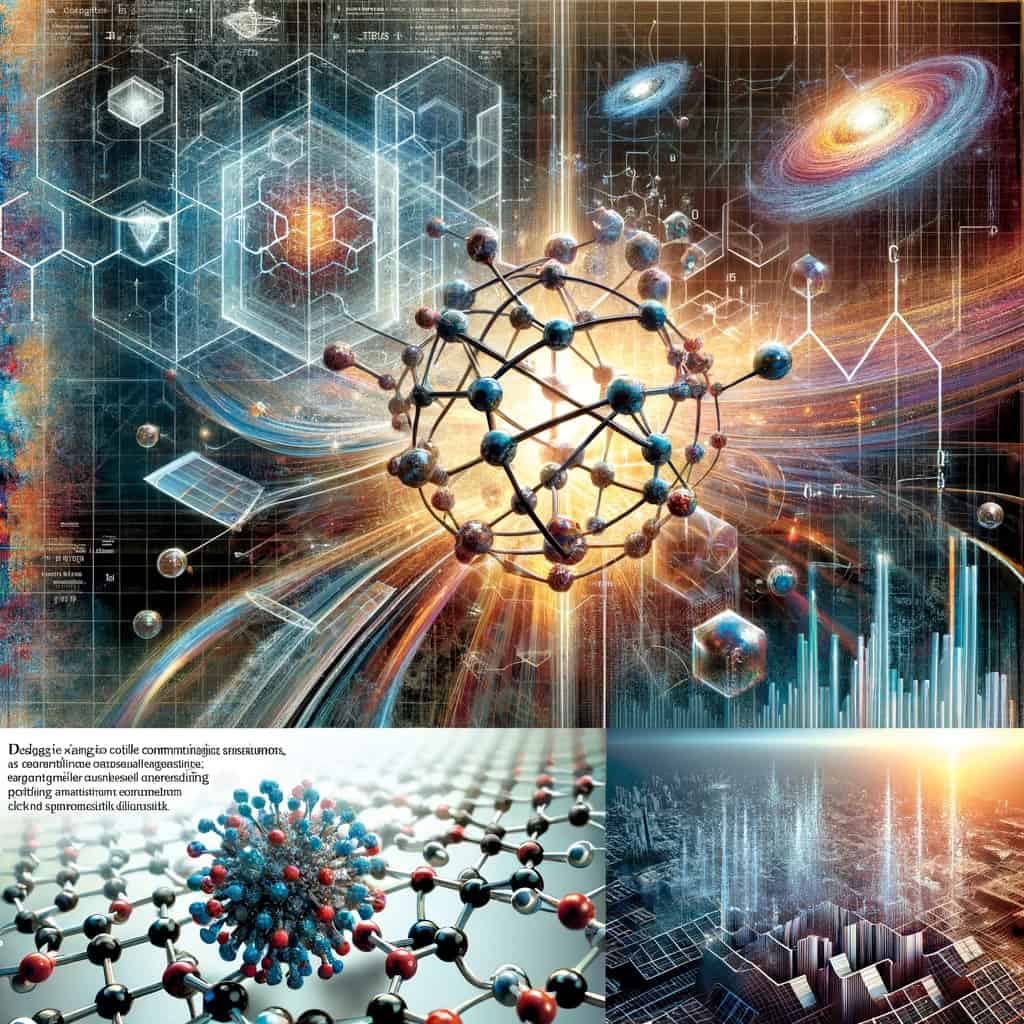
Introduction to Theoretical and Computational Chemistry
The area of theoretical and computational chemistry has progressed greatly over time, with computational chemistry as a discipline first emerging in the mid-1920s (Damm-Ganamet et al., 2020). Computational advances have improved the synergy between theory and practice in modern chemistry, transforming problem-solving approaches (Gatta et al., 2017). The incorporation of information technologies into education has also proven critical to increasing teaching efficacy, particularly in computational chemistry (Medetbayeva & Akhmetov, 2021).
Italy has made substantial contributions to theoretical and computational chemistry, with scholars making significant advances in a variety of molecular scientific domains (Barone, 2016). Theoretical and computational chemistry provide critical insights into molecular structures, characteristics, and reactivities, allowing us to better understand chemical systems and apply them to drug design and materials research (Lin, 2010; Morales-Navarro et al., 2019).
Computational chemistry has evolved by merging theoretical notions with experimental data, resulting in a thorough understanding of complicated chemical systems (Matta & Massa, 2011). High-quality electronic structure simulations, as well as the interpretative capacity of quantum mechanics, have played critical roles in the field’s advancement. Technological advancement has also played an important role, with exponential increases in processing capacity allowing academics to tackle increasingly complex problems (Gonçalves, 2019).
Theoretical chemistry has evolved into computational chemistry, with recent advances mainly reliant on computational methods (Truhlar, 2008). The use of computing power to solve theoretical equations and forecast chemical processes is becoming an essential component of modern chemistry research. The applications of theoretical and computational chemistry are broad, including medicinal chemistry, where computational methods have had a considerable impact on drug discovery and design (Satyanarayanajois & Hill, 2011).
The evolution of theoretical and computational chemistry has been marked by a symbiotic link between theory and practice, resulting in ground-breaking discoveries and novel applications across scientific fields. The combination of computational techniques and theoretical frameworks has improved our understanding of chemical systems and revolutionized problem-solving methodologies in chemistry.
Current Methods and Approaches
Current strategies in computational chemistry include a wide range of tools for accurately predicting chemical characteristics and reactions. Quantum mechanical simulations, molecular dynamics, and machine learning applications all play important roles in improving the area.
Quantum computing has emerged as a highly effective method for simulating complex chemical systems. Researchers have been investigating practical and massively parallel quantum computing emulation for quantum chemistry, harnessing the potential of quantum resources to simulate chemical and physical processes on a quantum scale. Shang et al. (2023; Aspuru-Guzik & Walther, 2012). Quantum algorithms have been developed for ground-state, dynamics, and thermal-state simulations, with advantages in precisely predicting chemical characteristics and reactions (Bauer et al., 2020). Quantum computers provide effective simulation of quantum systems, yielding insights into energy transfer and chemical isomerization processes (Lee et al., 2022; Straatsma & McCammon, 2001).
Molecular dynamics simulations, together with ab initio force fields, have proven useful in investigating molecular systems. These simulations, calibrated using cutting-edge computational approaches, allow for precise prediction of characteristics for molecules such as CH4, CCl4, CHF3, and CHCl3 dimers (Li et al., 2019). Researchers can do quantum molecular dynamics simulations by merging molecular dynamics with quantum mechanical/molecular mechanical simulations, resulting in a more thorough knowledge of chemical processes.
Machine learning applications have also transformed computational chemistry. Hybrid quantum-classical techniques have been developed to determine chemical systems’ ground or low energy states, increasing prediction accuracy (Lee et al., 2022). Quantum software programs based on the Full Quantum Eigensolver Algorithm have been proposed for chemistry simulations, delivering an exponential speedup over traditional optimization approaches (Li et al., 2022). Furthermore, quantum chemistry simulations in the seniority-zero space using qubit-based quantum computers have been refined, resulting in resource-efficient and highly accurate algorithms (Elfving et al., 2021).
The incorporation of quantum mechanical simulations, molecular dynamics, and machine learning applications into computational chemistry has greatly enhanced the science, allowing researchers to predict chemical characteristics and reactions with high precision. These technologies provide a view into the future of chemistry research, in which quantum computers and advanced simulation techniques will play a critical role in deciphering the intricacies of chemical systems.
Future Directions and Challenges
Emerging developments in theoretical and computational chemistry are determining the field’s future, with an emphasis on increasing computational power, improving algorithmic efficiency, and developing more accurate and predictive models. The combination of artificial intelligence (AI), quantum computing, and machine learning is transforming how chemical systems are researched and understood (Motta & Rice, 2021; Sheng & Zhang, 2012). These technologies have the potential to improve simulation accuracy and efficiency, allowing researchers to tackle more complicated systems and make more precise predictions about chemical characteristics.
Artificial intelligence is increasingly being used in chemistry to analyze large volumes of data, predict chemical attributes, and accelerate drug discovery (Motta & Rice, 2021). Machine learning algorithms are being developed to improve chemical reactions, create new materials, and forecast molecular behavior, providing important insights into chemical systems (Xu et al., 2021). The application of artificial intelligence in chemistry is likely to increase, with a focus on building more advanced algorithms to solve complex chemical issues.
Quantum computing is a significant leap in computational chemistry, with the potential for exponential speedups for handling complicated quantum mechanical issues (Motta & Rice, 2021). Quantum algorithms are being developed to simulate chemical reactions, forecast molecular characteristics, and optimize material design, opening the door to more accurate and efficient computational models (Motta & Rice, 2021). As quantum computing technology advances, it is projected to transform the study of theoretical and computational chemistry by providing simulations previously impossible with classical computers.
Emerging trends in computational chemistry include the development of sustainable battery chemistries, the investigation of new computational paradigms associated with exascale technologies, and the incorporation of molecular modeling into froth flotation studies (Felice, 2023; Sahraei et al., 2023; Andreadi et al., 2022). These developments indicate a growing emphasis on sustainability, efficiency, and innovation in computational techniques to analyzing chemical systems.
Scaling computations for complex systems continues to be a major concern for theoretical and computational chemistry researchers. As processing capacity increases, so is the demand for more efficient algorithms and software tools (Sahraei et al., 2023). Balancing the need for accuracy and speed in simulations, particularly for large-scale systems, is a key problem that necessitates continual R&D work (Sahraei et al., 2023; Asaduzzaman et al., 2019). Furthermore, maintaining the dependability and reproducibility of computational models and results is critical for progressing the science and understanding complicated chemical processes.
Finally, the future of theoretical and computational chemistry will be defined by the integration of cutting-edge technologies like as artificial intelligence, quantum computing, and machine learning to address difficult chemical problems and improve prediction skills. While obstacles in scaling computations and assuring correctness exist, constant research and innovation are propelling the area toward more efficient and trustworthy computational models.
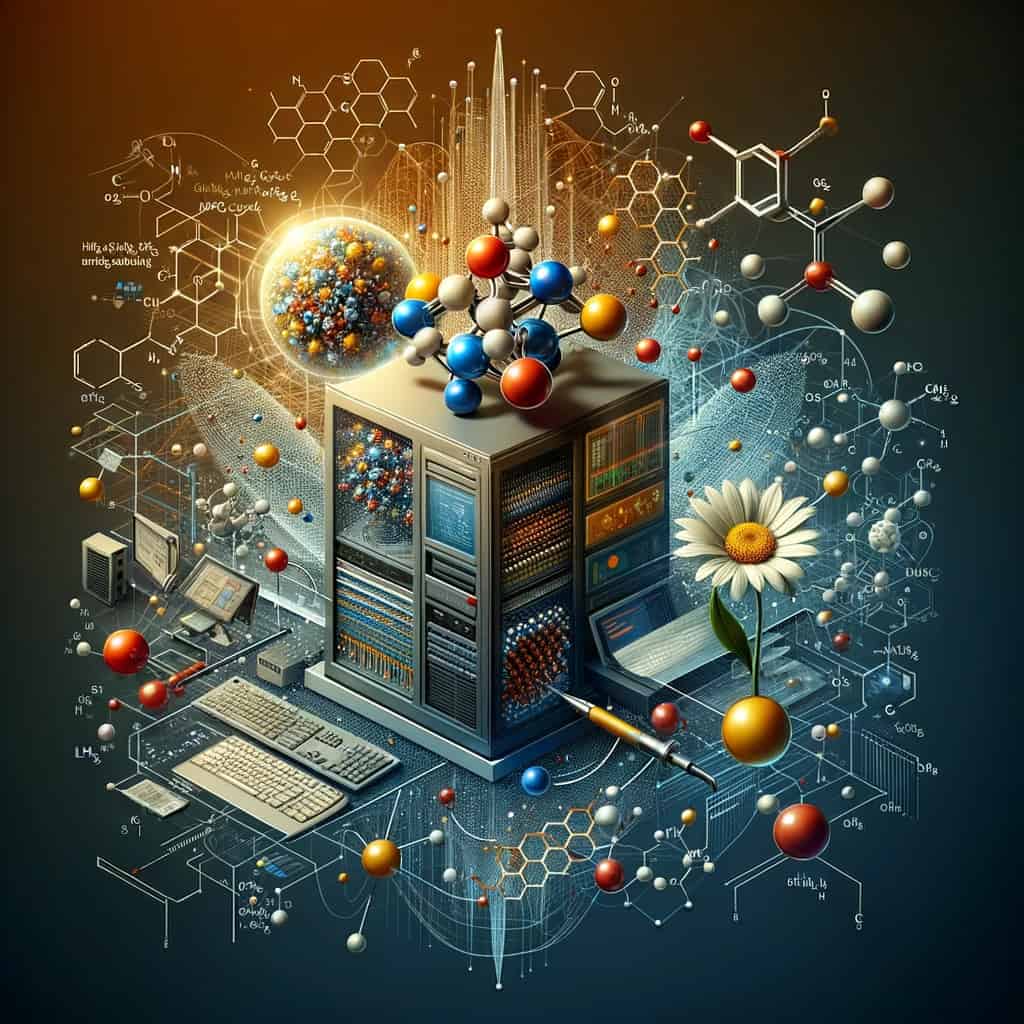
Reference:
Adhikari, J. and Adhikari, R. (2023). Free and open source codes for computational chemistry research initiation: a conspectus. Journal of Nepal Chemical Society, 43(2), 11-22. https://doi.org/10.3126/jncs.v43i2.53337
Andreadi, N., Zankov, D., Karpov, K., & Mitrofanov, A. (2022). Tree parzen estimator for global geometry optimization: a benchmark and database of experimental gas‐phase structures of organic molecules. Journal of Computational Chemistry, 43(21), 1434-1441. https://doi.org/10.1002/jcc.26947
Asaduzzaman, A., Riccardi, D., Afaneh, A., Cooper, C., Smith, J., Wang, F., … & Schreckenbach, G. (2019). Environmental mercury chemistry – in silico. Accounts of Chemical Research, 52(2), 379-388. https://doi.org/10.1021/acs.accounts.8b00454
Aspuru‐Guzik, A. and Walther, P. (2012). Photonic quantum simulators. Nature Physics, 8(4), 285-291. https://doi.org/10.1038/nphys2253
Barone, V. (2016). Theoretical and computational chemistry in italy. International Journal of Quantum Chemistry, 116(21), 1499-1500. https://doi.org/10.1002/qua.25291
Bauer, B., Bravyi, S., Motta, M., & Chan, G. (2020). Quantum algorithms for quantum chemistry and quantum materials science. Chemical Reviews, 120(22), 12685-12717. https://doi.org/10.1021/acs.chemrev.9b00829
Damm-Ganamet, K., DesJarlais, R., Marrone, T., Shih, A., Schiffer, J., Pérez-Benito, L., … & Mirzadegan, T. (2020). Breaking the glass ceiling in simulation and modeling: women in pharmaceutical discovery. Journal of Medicinal Chemistry, 63(5), 1929-1936. https://doi.org/10.1021/acs.jmedchem.9b01512
Elfving, V., Millaruelo, M., Gámez, J., & Gogolin, C. (2021). Simulating quantum chemistry in the seniority-zero space on qubit-based quantum computers. Physical Review A, 103(3). https://doi.org/10.1103/physreva.103.032605
Felice, R. (2023). A perspective on sustainable computational chemistry software development and integration. Journal of Chemical Theory and Computation, 19(20), 7056-7076. https://doi.org/10.1021/acs.jctc.3c00419
Gatta, G., Lotti, P., & Tabacchi, G. (2017). The effect of pressure on open-framework silicates: elastic behaviour and crystal–fluid interaction. Physics and Chemistry of Minerals, 45(2), 115-138. https://doi.org/10.1007/s00269-017-0916-z
Gonçalves, R. (2019). Overcoming frontiers of chemical reactions: reactive molecular dynamics simulations. Annals of Chemical Science Research, 1(2). https://doi.org/10.31031/acsr.2019.01.000508
Lee, C., Lau, J., Shi, L., & Kwek, L. (2022). Simulating energy transfer in molecular systems with digital quantum computers. Journal of Chemical Theory and Computation, 18(3), 1347-1358. https://doi.org/10.1021/acs.jctc.1c01296
Li, A., Wang, Y., & Chao, S. (2019). Molecular dynamics simulations with ab initio force fields: a review of case studies on ch4, ccl4, chf3, and chcl3 dimers. Multiscale Science and Engineering, 1(1), 26-33. https://doi.org/10.1007/s42493-018-00001-1
Li, H., Gao, P., Zhang, J., Liu, Z., Wei, H., Wen, K., … & Long, G. (2022). Bq-chem: a quantum software program for chemistry simulation based on the full quantum eigensolver algorithm. Quantum Engineering, 2022, 1-13. https://doi.org/10.1155/2022/5872283
Lin, Z. (2010). Interplay between theory and experiment: computational organometallic and transition metal chemistry. Accounts of Chemical Research, 43(5), 602-611. https://doi.org/10.1021/ar9002027
Matta, C. and Massa, L. (2011). Subsystem quantum mechanics and in silico medicinal and biological chemistry. Future Medicinal Chemistry, 3(16), 1971-1974. https://doi.org/10.4155/fmc.11.155
Medetbayeva, S. and Akhmetov, N. (2021). Psychological and pedagogical problems of computer-aided teaching of natural sciences. International Journal of Emerging Technologies in Learning (Ijet), 16(20), 208. https://doi.org/10.3991/ijet.v16i20.24427
Morales-Navarro, S., Prent-Peñaloza, L., Núñez, Y., Sánchez-Aros, L., Forero-Doria, O., González, W., … & Ramírez, D. (2019). Theoretical and experimental approaches aimed at drug design targeting neurodegenerative diseases. Processes, 7(12), 940. https://doi.org/10.3390/pr7120940
Motta, M. and Rice, J. (2021). Emerging quantum computing algorithms for quantum chemistry. Wiley Interdisciplinary Reviews Computational Molecular Science, 12(3). https://doi.org/10.1002/wcms.1580
Sahraei, A., Azizi, D., Mokarizadeh, A., Boffito, D., & Larachi, F. (2023). Emerging trends of computational chemistry and molecular modeling in froth flotation: a review. Acs Engineering Au, 3(3), 128-164. https://doi.org/10.1021/acsengineeringau.2c00053
Satyanarayanajois, S. and Hill, R. (2011). Medicinal chemistry for 2020. Future Medicinal Chemistry, 3(14), 1765-1786. https://doi.org/10.4155/fmc.11.135
Shang, H., Fan, Y., Shen, L., Guo, C., Liu, J., Duan, X., … & Li, Z. (2023). Towards practical and massively parallel quantum computing emulation for quantum chemistry. NPJ Quantum Information, 9(1). https://doi.org/10.1038/s41534-023-00696-7
Sheng, C. and Zhang, W. (2012). Fragment informatics and computational fragment‐based drug design: an overview and update. Medicinal Research Reviews, 33(3), 554-598. https://doi.org/10.1002/med.21255
Straatsma, T. and McCammon, J. (2001). Load balancing of molecular dynamics simulation with nwchem. Ibm Systems Journal, 40(2), 328-341. https://doi.org/10.1147/sj.402.0328
Truhlar, D. (2008). Molecular modeling of complex chemical systems. Journal of the American Chemical Society, 130(50), 16824-16827. https://doi.org/10.1021/ja808927h
Xu, P., Tan, D., & Chen, Z. (2021). Emerging trends in sustainable battery chemistries. Trends in Chemistry, 3(8), 620-630. https://doi.org/10.1016/j.trechm.2021.04.007