Beyond AlphaFold 3: Navigating Future Challenges in Protein Structure Prediction
AlphaFold 3, developed by Google DeepMind, is a game-changing development in predicting the structure and interactions of biomolecules. This model, published in Nature, considerably improves the ability to model proteins, DNA, RNA, and other molecules, outperforming existing techniques for protein interactions by at least 50%.
This study examines current advances in protein structure prediction, focusing on computational approaches, their applications in drug development, and the emerging role of artificial intelligence in improving predicted accuracy.
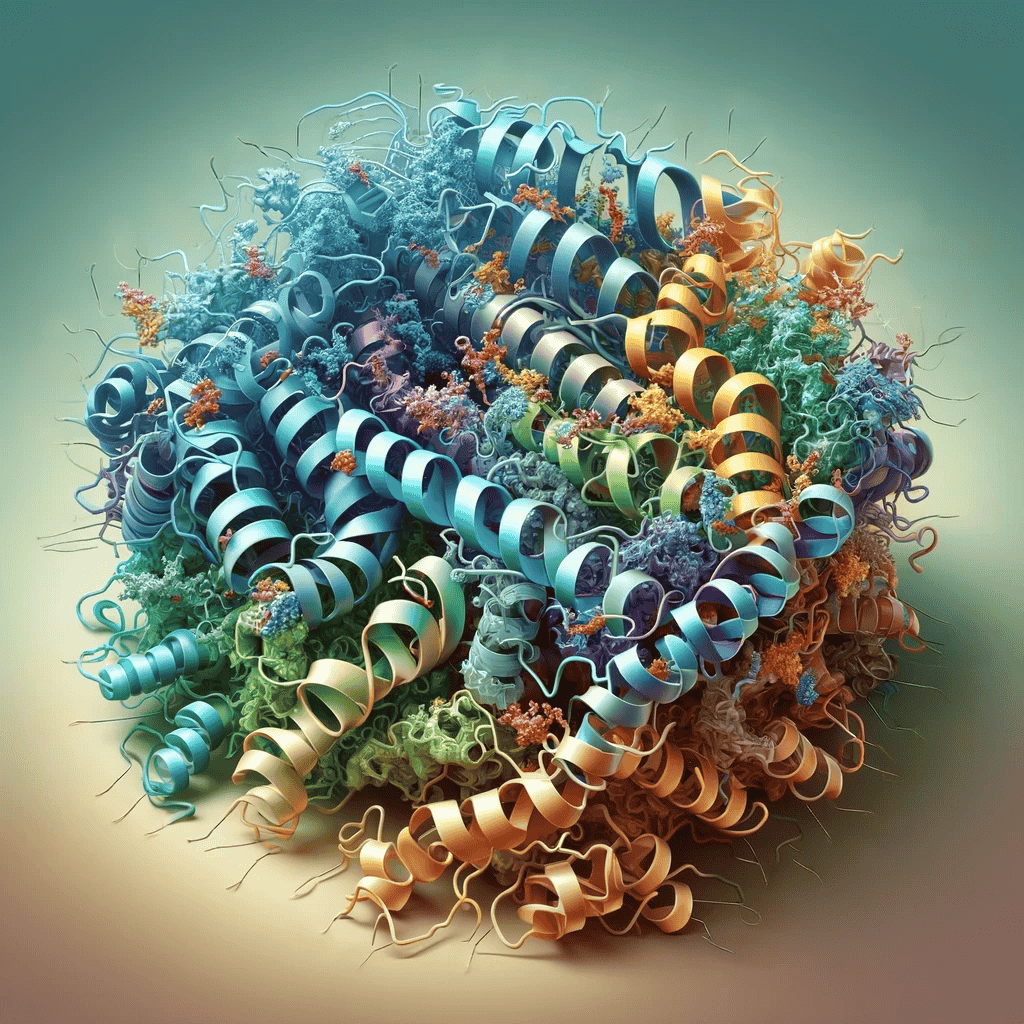
Introduction to Protein Structure Prediction
Proteins are vital molecules in living organisms, and their functions are inextricably tied to their three-dimensional shapes. Predicting protein structures is critical for understanding their activities, interactions, and roles in biological processes. Protein structure prediction has advanced significantly, with many computational approaches created to decode protein structures from amino acid sequences.
Protein structure prediction has traditionally proved difficult, as evidenced by the Critical Assessment of Protein Structure Prediction (CASP) tests (Khor et al., 2015). These trials were significant in determining the accuracy and dependability of various prediction systems. Protein structure prediction approaches are generically classified as homology modeling, threading, and ab initio modeling, based on the availability of solved structures (Pi & Sael, 2013). According to Dapkūnas et al. (2019), template-based modeling, or homology modeling, is the most reliable method for predicting protein structures.
Recent advances in the field, such as the creation of neural network-based techniques like the three-track network, have yielded encouraging results in accurately predicting protein shapes and interactions (Baek et al., 2021). These strategies aided in the speedy resolution of difficult structure modeling challenges and provided insights into the functions of proteins with unknown structures. Furthermore, deep learning techniques have led to advances in predicting intra-protein interactions, improving the accuracy of structure prediction tasks such as CASP (Yan & Huang, 2021).
Despite these advances, obstacles remain in the field of protein structure prediction. The limits of existing bioinformatic methods make accurately predicting membrane protein structures a considerable difficulty (Gao & Cross, 2005). Furthermore, modern approaches such as AlphaFold still struggle to reliably anticipate minor structural changes caused by single-site mutations in protein sequences (Mahmud, 2023).
Protein structure prediction is essential for understanding protein activities and interactions. While substantial work has been made in creating computational approaches for structure prediction, problems remain, such as predicting membrane protein structures and effectively capturing minor structural changes. Continued research and advances in computational biology and artificial intelligence are critical to enhancing the accuracy and reliability of protein structure prediction systems.
Methods of Protein Structure Prediction
Protein structure prediction uses a variety of computational approaches, including homology modeling, ab initio methods, and machine learning techniques. Homology modeling is a popular method for predicting protein structures based on known homologs (Muhammed & Akı-Yalçın, 2018). This approach is useful for drug development and protein function analysis (Muhammed & Akı-Yalçın, 2018). Ab initio approaches, on the other hand, seek to predict protein structures exclusively from amino acid sequences rather than relying on known structures (Kong et al., 2021). While ab initio approaches are powerful, they are computationally expensive and less accurate than homology modeling when appropriate templates are unavailable (Kong et al., 2021).
Machine learning, particularly deep learning approaches, has made substantial advances in protein structure prediction. Deep learning algorithms, such as neural networks, have demonstrated extraordinary accuracy in predicting protein shapes and interactions. These techniques use massive volumes of data to discover intricate patterns and correlations within protein sequences and structures, resulting in higher prediction accuracy. Deep learning, for example, has been useful in accurately identifying intra-protein interactions, hence improving overall structure prediction quality.
A comparative examination of various methods reveals that homology modeling is extremely effective when sufficient templates are available, providing solid predictions for a wide range of proteins. While computationally intensive, ab initio approaches are useful for predicting novel protein structures in the absence of homologous templates (Kong et al., 2021). Machine learning approaches, particularly deep learning, have shown considerable promise in improving the accuracy and efficiency of protein structure prediction, particularly in capturing complex structural characteristics and interactions.
Protein structure prediction methods, such as homology modeling, ab initio approaches, and machine learning techniques, each have advantages and drawbacks. Recent advances in deep learning have considerably increased the accuracy and reliability of protein structure prediction, opening the door to more exact modeling of complicated protein structures and interactions.
Applications and Future Directions
Accurate protein structure prediction is critical for drug development and molecular biology, as it provides information on protein activities and interactions. Homology modeling, a computational method based on evolutionary conservation, is widely used in drug development to predict protein structures when sufficient templates are available. Muhammad and Akı-Yalçın (2018). This technique helps to understand pharmacological mechanisms of action and build compounds that selectively bind to target proteins (Saha et al., 2018). Accurate protein structure prediction is crucial for rational drug design, estimating binding free energies and promoting structure-based drug discovery (Özçelik et al., 2023).
Emerging technologies, such as deep learning and artificial intelligence (AI), have altered protein structure predictions and drug discovery. Deep learning algorithms, such as AlphaFold, have considerably increased protein structure prediction accuracy, allowing for high-throughput structure-based drug discovery and functional analysis (Zheng et al., 2022). These developments have the potential to speed up drug discovery by giving precise structural information about target proteins (Meller et al., 2023). Integrative techniques that combine machine learning and physics-based scoring systems provide potential for improving drug discovery outcomes by accurately predicting protein-ligand binding affinities (Guedes et al., 2021).
Future research in protein structure prediction and drug discovery is likely to focus on overcoming the difficulties of reliably predicting protein-ligand binding sites in order to facilitate computationally driven drug discovery programs (Beuming et al.,2022). AI’s evolving involvement in protein structure prediction is projected to shape the area further, with AI-based methodologies becoming more trustworthy and beneficial for small-molecule drug development initiatives (Yin et al., 2021). Cryo-electron microscopy, when paired with AI modeling tools, is expected to play an increasingly important role in computational drug design, improving the accuracy and utility of drug discovery procedures (Kim & Lee, 2020).
To summarize, accurate protein structure prediction is critical for developing drug discovery and molecular biology. The incorporation of emerging technologies, such as deep learning and AI, has the potential to increase the accuracy and efficiency of protein structure prediction, ultimately leading to better drug discovery results.
References
Baek, M., DiMaio, F., Anishchenko, I., Dauparas, J., Овчинников, С., Lee, G., … & Baker, D. (2021). Accurate prediction of protein structures and interactions using a three-track neural network. Science, 373(6557), 871-876. https://doi.org/10.1126/science.abj8754
Dapkūnas, J., Olechnovič, K., & Venclovas, C. (2019). Structural modeling of protein complexes: current capabilities and challenges. Proteins Structure Function and Bioinformatics, 87(12), 1222-1232. https://doi.org/10.1002/prot.25774
Gao, F. and Cross, T. (2005). Untitled. Genome Biology, 6(13), 244. https://doi.org/10.1186/gb-2005-6-13-244
Khor, B., Tye, G., Lim, T., & Choong, Y. (2015). General overview on structure prediction of twilight-zone proteins. Theoretical Biology and Medical Modelling, 12(1). https://doi.org/10.1186/s12976-015-0014-1
Mahmud, S. (2023). Accurate prediction of protein tertiary structural changes induced by single-site mutations with equivariant graph neural networks.. https://doi.org/10.1101/2023.10.03.560758
Pi, J. and Sael, L. (2013). Mass spectrometry coupled experiments and protein structure modeling methods. International Journal of Molecular Sciences, 14(10), 20635-20657. https://doi.org/10.3390/ijms141020635
Yan, Y. and Huang, S. (2021). Accurate prediction of inter-protein residue–residue contacts for homo-oligomeric protein complexes. Briefings in Bioinformatics, 22(5). https://doi.org/10.1093/bib/bbab038
Kong, L., Ju, F., Zhang, H., Sun, S., & Bu, D. (2021). Falcon2: a web server for high-quality prediction of protein tertiary structures. BMC Bioinformatics, 22(1). https://doi.org/10.1186/s12859-021-04353-8
Muhammed, M. and Akı-Yalçın, E. (2018). Homology modeling in drug discovery: overview, current applications, and future perspectives. Chemical Biology & Drug Design, 93(1), 12-20. https://doi.org/10.1111/cbdd.13388
Beuming, T., Martín, H., Díaz-Rovira, A., Díaz, L., Guallar, V., & Ray, S. (2022). Are deep learning structural models sufficiently accurate for free energy calculations? application of fep+ to alphafold2 predicted structures.. https://doi.org/10.1101/2022.08.16.504122
Guedes, I., Barreto, A., Marinho, D., Krempser, E., Kuenemann, M., Spérandio, O., … & Miteva, M. (2021). New machine learning and physics-based scoring functions for drug discovery. Scientific Reports, 11(1). https://doi.org/10.1038/s41598-021-82410-1
Kim, J. and Lee, J. (2020). Cryo-electron microscopy research at the institute of membrane proteins: current status and future prospects. Korean Society for Structural Biology, 8(4), 87-92. https://doi.org/10.34184/kssb.2020.8.4.87
Meller, A., Oliviera, S., Abramyan, T., Davtyan, A., Bowman, G., & Bedem, H. (2023). Discovery of a cryptic pocket in the ai-predicted structure of ppm1d phosphatase explains the binding site and potency of its allosteric inhibitors.. https://doi.org/10.1101/2023.03.22.533829
Muhammed, M. and Akı-Yalçın, E. (2018). Homology modeling in drug discovery: overview, current applications, and future perspectives. Chemical Biology & Drug Design, 93(1), 12-20. https://doi.org/10.1111/cbdd.13388
Saha, A., Shih, A., Mirzadegan, T., & Seierstad, M. (2018). Predicting the binding of fatty acid amide hydrolase inhibitors by free energy perturbation. Journal of Chemical Theory and Computation, 14(11), 5815-5822. https://doi.org/10.1021/acs.jctc.8b00672
Yin, J., Yu, J., Cui, W., Lei, J., Chen, W., Satz, A., … & Kuai, L. (2021). Assessment of ai-based protein structure prediction for the nlrp3 target.. https://doi.org/10.1101/2021.11.05.467381
Zheng, L., Meng, J., Jiang, K., Lan, H., Wang, Z., Lin, M., … & Mu, Y. (2022). Improving protein–ligand docking and screening accuracies by incorporating a scoring function correction term. Briefings in Bioinformatics, 23(3). https://doi.org/10.1093/bib/bbac051
Özçelik, R., Tilborg, D., Jiménez-Luna, J., & Grisoni, F. (2023). Structure‐based drug discovery with deep learning**. Chembiochem, 24(13). https://doi.org/10.1002/cbic.202200776