Accelerating Chemical Innovation with Transfer Learning
The technique of transfer learning has become very important in the area of chemistry, making research much more efficient and useful. Researchers can cut down on training costs, get better predictions of properties, and make molecular design easier by using models that have already been taught. This collection of information is especially useful for reusing data, adapting to new domains, and improving performance in a wide range of chemical jobs.
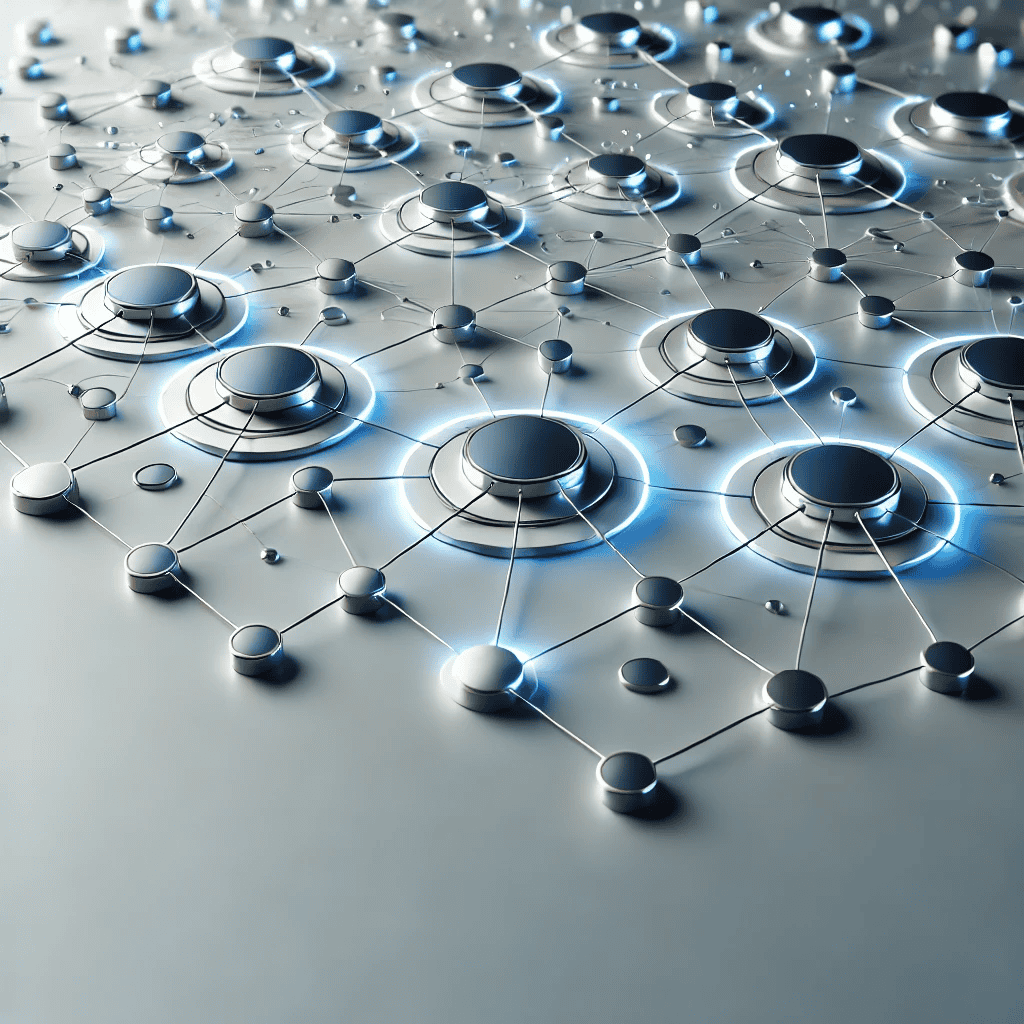
One of the best things about transfer learning in chemistry is that it can lower the cost of training. With small datasets, pre-trained models can be fine-tuned for specific chemical tasks. These models can be based on deep learning designs like BERT and graph neural networks (GNNs). For instance, YieldBERT utilizes a pre-trained BERT encoder to predict chemical reaction yields, showing how existing models can be adapted for new applications without the need for extensive retraining (Shi, 2024). According to Han (2024), GNNs can improve the accuracy of predictions for chemical reaction rates by starting models with weights that have already been trained. This is done by using representations that have already been learned. This method not only saves computer power but also speeds up research by letting scientists focus on making predictions better instead of starting from scratch with models.
Transfer learning also makes domain adaptation easier, which means that models that were learned on one type of chemical data can be used effectively on another. For instance, Sekeran et al. showed how a model learned on one type of battery cell chemistry could be used to predict the end-of-life of a different type of chemistry. This showed how transfer learning can be used to deal with a wide range of chemical systems (Sekeran et al., 2022). This flexibility is very important in chemistry, where labeled data isn’t always easy to find. Being able to generalize across different fields can greatly improve the results of study.
Transfer learning improves performance, which can be seen in a number of chemical jobs. Studies have shown that models that use transfer learning always do better than models that were only taught on task-specific data. For example, Wu et al. showed that transfer learning works well in situations with few resources, where standard data-driven methods fail because there isn’t enough training data (Wu et al., 2022). Using advanced molecular representations, like those from variational autoencoders (VAEs) and geometry-enhanced learning methods, also makes the model work better by showing how molecular structures and properties are connected in very complex ways (Wigh et al., 2022; Fang et al., 2022). These improvements show how important it is to combine transfer learning with advanced molecular representation methods to get better prediction abilities.
Transfer learning is changing chemical research by letting data be used again and again, making it easier to adapt to new domains, and improving success on many tasks. Using models that have already been trained not only cuts down on the cost of training but also speeds up the process of finding new things in chemistry. Transfer learning combined with new modeling methods is likely to lead to even bigger progress as the field continues to change. This will make chemical study more efficient and useful.
References
Fang, X., Liu, L., Lei, J., He, D., Zhang, S., Zhou, J., … & Wang, H. (2022). Geometry-enhanced molecular representation learning for property prediction. Nature Machine Intelligence, 4(2), 127-134. https://doi.org/10.1038/s42256-021-00438-4
Han, J. (2024). Improving chemical reaction yield prediction using pre-trained graph neural networks. Journal of Cheminformatics, 16(1). https://doi.org/10.1186/s13321-024-00818-z
Sekeran, M., Živadinović, M., & Spiliopoulou, M. (2022). Transferability of a battery cell end-of-life prediction model using survival analysis. Energies, 15(8), 2930. https://doi.org/10.3390/en15082930
Shi, R. (2024). Prediction of chemical reaction yields with large-scale multi-view pre-training. Journal of Cheminformatics, 16(1). https://doi.org/10.1186/s13321-024-00815-2
Wigh, D., Goodman, J., & Lapkin, A. (2022). A review of molecular representation in the age of machine learning. Wiley Interdisciplinary Reviews Computational Molecular Science, 12(5). https://doi.org/10.1002/wcms.1603
Wu, Z., Cai, X., Zhang, C., Qiao, H., Wu, Y., Zhang, Y., … & Duan, H. (2022). Self-supervised molecular pretraining strategy for low-resource reaction prediction scenarios. Journal of Chemical Information and Modeling, 62(19), 4579-4590. https://doi.org/10.1021/acs.jcim.2c00588