Integration of Machine Learning with Chemistry
Machine learning enhances various fields by improving predictions and facilitating discoveries, especially in chemistry, drug development, and materials science.
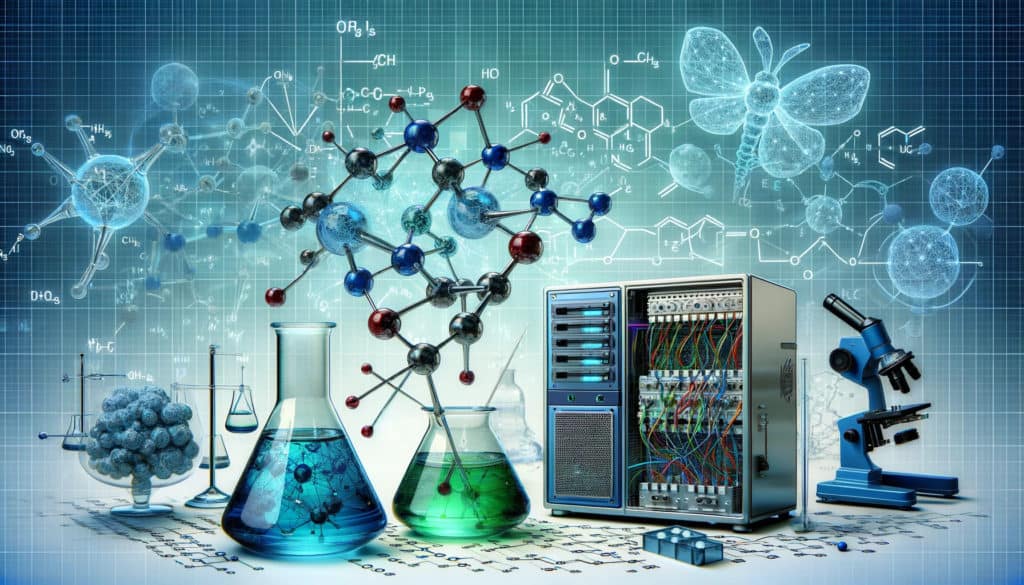
1 Principles and Approaches of Machine Learning in Chemistry
Machine learning has had a profound effect on the chemistry profession by allowing computers to learn from data and solve intricate issues (Mater & Coote, 2019). ML approaches have been increasingly incorporated into chemistry, especially in drug discovery and computational chemistry. Deep learning, a subset of machine learning, has become more popular because of its distinctive characteristics that set it apart from conventional machine learning algorithms (Goh et al., 2017). Deep neural networks have been successfully used in cheminformatics to create strong models for drug discovery and chemical research (Pfau et al., 2020).
ML approaches like supervised and unsupervised learning, deep learning, and reinforcement learning have been essential in addressing chemical issues. The methods have played a crucial role in predicting molecular characteristics, bioactivity, and molecular structures with precision (Tilborg et al., 2022). Generative models have been effectively used in drug development to produce new chemical compounds with desired characteristics, showing encouraging outcomes (Bian & Xie, 2021).
Data collection for machine learning applications in chemistry includes sources like experimental data, computational simulations, and databases. Preprocessing this data is challenging since it is complicated and heterogeneous. Advances in data augmentation and transfer learning techniques have improved the ability to forecast chemical reactions, benefiting organic chemistry and drug development research (Zhang et al., 2021). Feature representation and selection are crucial in chemistry to create precise machine learning models. Chemical descriptors, fingerprints, and other feature representations are essential in training models for predicting molecular properties and behaviors (Korshunova et al., 2021).
The use of machine learning in chemistry has merged with quantum chemistry, allowing for extensive exploration of chemical space by quantum calculations (Schütt et al., 2019). Deep learning architectures have improved the prediction of chemical patterns, leading to better knowledge and prediction of chemical phenomena (Cova & Pais, 2019). ML has been utilized in designing covalent protein kinase inhibitors and predicting solvation free energy, demonstrating its adaptability in several areas of chemistry (Yoshimori et al., 2022; Yu et al., 2023).
Ultimately, the integration of machine learning in the field of chemistry has greatly influenced research and advancement in drug discovery, computational chemistry, and materials science. Deep learning methods, along with improvements in data preprocessing and feature representation, have advanced the area by enabling more precise predictions and groundbreaking discoveries.
2 Applications of Machine Learning in Chemistry
Machine learning has greatly influenced drug discovery and development by transforming the forecasting of chemical characteristics, drug-likeness, and bioactivity (Schütt et al., 2019). OpenChem provides advanced deep learning features for computational chemistry and drug discovery, improving the accuracy of forecasting chemical characteristics (Korshunova et al., 2021). Graph machine learning models in environmental chemistry have been essential for forecasting environmental qualities, aiding in sustainable development (Zhu et al., 2023).
Kernel-based learning approaches in drug design are useful for classifying drug-likeness and identifying compounds with desirable features (Müller et al., 2005). Machine learning methods have been effectively combined with molecular dynamics simulations to estimate binding affinities important for drug development (Riniker et al., 2019). Transfer learning has enhanced interatomic neural network potentials, leading to improved accuracy in forecasting physical and chemical phenomena in materials science (Zaverkin et al., 2023).
The references highlight the various uses of machine learning in chemistry, ranging from medication design to environmental chemistry. ML techniques have improved predictive modeling and aided in discovering new materials, enhancing material synthesis processes, and deepening the understanding of chemical reactions in several chemistry fields.
3 Challenges, Constraints, and Prospects for the Future
3.1 Obstacles and Constraints in Combining Machine Learning with Chemistry:
Integrating machine learning with chemistry encounters hurdles such as data quality and availability, model interpretability, and the requirement for domain expertise (Cova & Pais, 2019). Addressing challenges such as cleaning data, assuring accuracy and impartiality in chemical information generation, standardizing chemical data, and promoting collaboration opportunities are essential obstacles that must be resolved (Cova & Pais, 2019). The chemistry sector faces a significant difficulty due to the lack of expertise and familiarity with machine learning (ML) and deep learning. This highlights the relevance of domain knowledge in effectively employing ML approaches in chemical research (Cova & Pais, 2019).
3.2 Advancements in Algorithmic Approaches for Chemical Applications:
Recent progress in machine learning algorithms has greatly improved their usefulness in chemical applications. Graph neural networks and transfer learning have demonstrated potential in overcoming obstacles in chemical research (Artrith et al., 2021). The algorithmic methods have improved the way machine learning models represent, withstand challenges, and apply to various situations, leading to more effective and precise forecasts in the field of chemistry (Artrith et al., 2021).
3.3 Integrating Experimental and Computational Chemistry:
Efforts to combine machine learning with both experimental and computational chemistry have been crucial in promoting a comprehensive approach to chemical research (Joshi & Kumar, 2021). Researchers can expand their understanding of chemical events and optimize research outcomes by integrating experimental data with computer models. This integration allows for the creation of more precise predictive models and promotes progress in several fields of chemistry.
3.4 Future Prospects of Integrating Machine Learning with Chemistry:
In the future, combining quantum computing with machine learning has the potential to significantly transform chemical research (Huang et al., 2022). Quantum computing can greatly increase computational capacity for intricate chemical simulations and predictions, resulting in advancements in drug discovery, materials science, and environmental chemistry. Additionally, the implementation of machine learning-powered robotics to automate laboratory procedures and the creation of universal chemical predictors are projected to enhance research efficiency and speed up advancements in the chemistry sector (Langs et al., 2018).
Overall, despite existing obstacles in combining machine learning with chemistry, recent progress in algorithms, initiatives to connect experimental and computational chemistry, and upcoming developments like quantum computing and automation offer promising chances for revolutionary advancements in chemical research.
References:
Artrith, N., Butler, K., Coudert, F., Han, S., Isayev, O., Jain, A., … & Walsh, A. (2021). Best practices in machine learning for chemistry. Nature Chemistry, 13(6), 505-508. https://doi.org/10.1038/s41557-021-00716-z
Bian, Y. and Xie, X. (2021). Generative chemistry: drug discovery with deep learning generative models. Journal of Molecular Modeling, 27(3). https://doi.org/10.1007/s00894-021-04674-8
Cova, T. and Pais, A. (2019). Deep learning for deep chemistry: optimizing the prediction of chemical patterns. Frontiers in Chemistry, 7. https://doi.org/10.3389/fchem.2019.00809
Cova, T. and Pais, A. (2019). Deep learning for deep chemistry: optimizing the prediction of chemical patterns. Frontiers in Chemistry, 7. https://doi.org/10.3389/fchem.2019.00809
Goh, G., Hodas, N., & Vishnu, A. (2017). Deep learning for computational chemistry. Journal of Computational Chemistry, 38(16), 1291-1307. https://doi.org/10.1002/jcc.24764
Huang, H., Kueng, R., Torlai, G., Albert, V., & Preskill, J. (2022). Provably efficient machine learning for quantum many-body problems. Science, 377(6613). https://doi.org/10.1126/science.abk3333
Joshi, R. and Kumar, N. (2021). Artificial intelligence for autonomous molecular design: a perspective. Molecules, 26(22), 6761. https://doi.org/10.3390/molecules26226761
Korshunova, M., Ginsburg, B., Tropsha, A., & Isayev, O. (2021). Openchem: a deep learning toolkit for computational chemistry and drug design. Journal of Chemical Information and Modeling, 61(1), 7-13. https://doi.org/10.1021/acs.jcim.0c00971
Korshunova, M., Ginsburg, B., Tropsha, A., & Isayev, O. (2021). Openchem: a deep learning toolkit for computational chemistry and drug design. Journal of Chemical Information and Modeling, 61(1), 7-13. https://doi.org/10.1021/acs.jcim.0c00971
Langs, G., Röhrich, S., Hofmanninger, J., Prayer, F., Pan, J., Herold, C., … & Prosch, H. (2018). Machine learning: from radiomics to discovery and routine. Der Radiologe, 58(S1), 1-6. https://doi.org/10.1007/s00117-018-0407-3
Mater, A. and Coote, M. (2019). Deep learning in chemistry. Journal of Chemical Information and Modeling, 59(6), 2545-2559. https://doi.org/10.1021/acs.jcim.9b00266
Müller, K., Rätsch, G., Sonnenburg, S., Mika, S., Grimm, M., & Heinrich, N. (2005). Classifying ‘drug-likeness’ with kernel-based learning methods. Journal of Chemical Information and Modeling, 45(2), 249-253. https://doi.org/10.1021/ci049737o
Pfau, D., Spencer, J., Matthews, A., & Foulkes, W. (2020). ab initiosolution of the many-electron schrödinger equation with deep neural networks. Physical Review Research, 2(3). https://doi.org/10.1103/physrevresearch.2.033429
Riniker, S., Wang, S., Bleiziffer, P., Böselt, L., & Esposito, C. (2019). Machine learning with and for molecular dynamics simulations. Chimia International Journal for Chemistry, 73(12), 1024. https://doi.org/10.2533/chimia.2019.1024
Schütt, K., Gastegger, M., Tkatchenko, A., Müller, K., & Maurer, R. (2019). Unifying machine learning and quantum chemistry with a deep neural network for molecular wavefunctions. Nature Communications, 10(1). https://doi.org/10.1038/s41467-019-12875-2
Schütt, K., Gastegger, M., Tkatchenko, A., Müller, K., & Maurer, R. (2019). Unifying machine learning and quantum chemistry with a deep neural network for molecular wavefunctions. Nature Communications, 10(1). https://doi.org/10.1038/s41467-019-12875-2
Tilborg, D., Alenicheva, A., & Grisoni, F. (2022). Exposing the limitations of molecular machine learning with activity cliffs. Journal of Chemical Information and Modeling, 62(23), 5938-5951. https://doi.org/10.1021/acs.jcim.2c01073
Yoshimori, A., Miljković, F., & Bajorath, J. (2022). Approach for the design of covalent protein kinase inhibitors via focused deep generative modeling. Molecules, 27(2), 570. https://doi.org/10.3390/molecules27020570
Yu, J., Zhang, C., Cheng, Y., Yang, Y., She, Y., Liu, F., … & Su, A. (2023). Solvbert for solvation free energy and solubility prediction: a demonstration of an nlp model for predicting the properties of molecular complexes. Digital Discovery, 2(2), 409-421. https://doi.org/10.1039/d2dd00107a
Zaverkin, V., Holzmüller, D., Bonfirraro, L., & Kästner, J. (2023). Transfer learning for chemically accurate interatomic neural network potentials. Physical Chemistry Chemical Physics, 25(7), 5383-5396. https://doi.org/10.1039/d2cp05793j
Zhang, Y., Wang, X., Zhang, C., Ge, J., Tang, J., Su, A., … & Duan, H. (2021). Data augmentation and transfer learning strategies for reaction prediction in low chemical data regimes. Organic Chemistry Frontiers, 8(7), 1415-1423. https://doi.org/10.1039/d0qo01636e
Zhu, S., Nguyen, B., Xia, Y., Frost, K., Xie, S., Viswanathan, V., … & Smith, J. (2023). Improved environmental chemistry property prediction of molecules with graph machine learning. Green Chemistry, 25(17), 6612-6617. https://doi.org/10.1039/d3gc01920a